Einstein Conversation Mining is an AI-driven tool that helps businesses analyze customer interactions across various communication channels, such as emails, chat, and call transcripts. It enables companies to gain deeper insights into customer needs, improve service, and optimize sales strategies by identifying trends, sentiment, and key topics.
However, Salesforce Einstein Conversation Mining requires proper training to deliver accurate and actionable insights. Well-structured AI training ensures the system recognizes patterns effectively, reducing errors and improving the quality of sentiment analysis, sales forecasting, and process automation. Let’s explore the best practices to train Einstein Conversation Mining for maximum accuracy and efficiency.
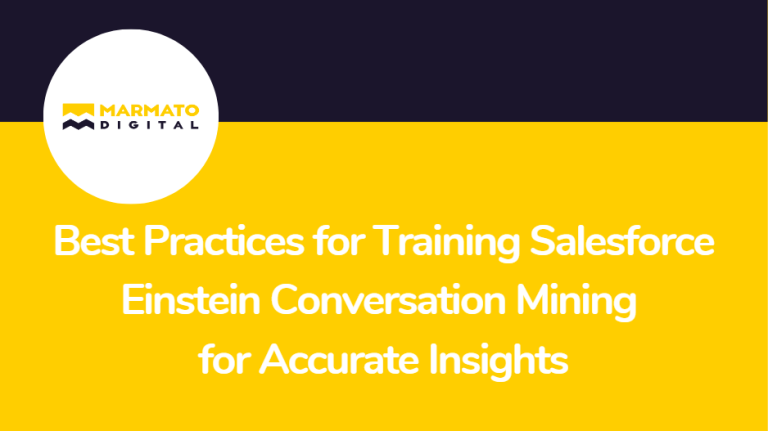
How Salesforce Einstein Conversation Mining Works?
Einstein Conversation Mining leverages Natural Language Processing (NLP) and machine learning to analyze unstructured text data from customer interactions. The AI model processes conversations to detect key topics, classify intent, and extract actionable insights.
Key Components:
- Text Processing: The AI scans emails, chat transcripts, and call logs to identify patterns.
- Machine Learning: It learns from historical data to improve accuracy over time.
- Labeling & Training: Fine-tuning the AI with labeled data enhances its ability to recognize specific business terms and customer concerns.
Best Practices for Training Einstein Conversation Mining:
A. Defining Clear Business Objectives:
Before training the AI, it’s essential to establish what insights you want to gain. Align AI training with key performance indicators (KPIs) related to marketing, sales, or customer service.
Clearly define objectives such as:
- Identifying customer pain points from conversations.
- Tracking sentiment trends for better customer experience management.
- Detecting common sales objections to refine sales strategies.
B. Collecting and Preparing High-Quality Training Data:
- Use Diverse Datasets: Include conversations from various customer segments and languages.
- Clean and Preprocess Data: Remove typos, irrelevant text, and excessive jargon.
- Ensure Balanced Representation: Include positive, neutral, and negative interactions for a well-rounded training dataset.
C. Leveraging Human-AI Collaboration for Labeling:
- Use Human Review: Employ human-in-the-loop (HITL) methods to review and label data manually.
- Set Up a Feedback Loop: Refine AI predictions based on human validation.
- Identify Misclassifications: Train teams to recognize edge cases and adjust models accordingly.
D. Fine-Tuning AI Models for Greater Accuracy:
- Adjust keyword matching and sentiment analysis thresholds to minimize false positives and negatives.
- Use custom training models tailored to industry-specific terminology.
- Retrain models periodically to adapt to evolving customer conversations.
E. Monitoring and Measuring Model Performance:
- Track key AI performance metrics such as precision, recall, and F1-score.
- Conduct A/B testing to compare AI-generated insights with human analysis.
- Establish a continuous validation process where customer support or sales teams review AI predictions.
F. Optimize Data Segmentation and Filtering:
- Filter Out Irrelevant Data: Ensure only free-text inputs are analyzed.
- Use High-Quality Data: Prioritize real customer interactions from Email-to-Case, Chat, and Voice.
- Exclude Duplicates and Noise: Avoid redundant reports with the same filters, as clustering algorithms may yield different results.
G. Improve Language and Regional Accuracy:
- Select Supported Languages: Einstein Conversation Mining officially supports English, French, German, Italian, and Spanish.
- Enable Generative AI for Multilingual Support: If working with additional languages, enable Generative AI in Salesforce.
- Account for Dialect and Regional Variations: Adjust AI models to reflect regional language nuances.
H. Ensure Effective Report Structuring:
- Use the Right Data Sources: For Email-to-Case reports, ensure emails are linked to a Case (ParentId field = Case).
- Leverage Web-to-Case for Free-Text Fields: Avoid analyzing non-free-text fields.
- Understand Report Limitations: Note that Enhanced Conversations aren’t supported in sandboxes, and Web-to-Case reports aren’t included in Service Intelligence dashboards.
I. Improve Clustering and Topic Accuracy:
- Refine Report Filters for Consistency: Since clustering algorithms generate slightly different results, use consistent filters across reports.
- Monitor Contact Reason Excerpts: Be aware of limits: 500 excerpts per conversation reason (or 3,000 excerpts when using Data Cloud API).
- Use the Transcript Record Count: To determine the volume of data analyzed, check Transcript Record Count, not just conversation excerpts.
J. Enhance AI Training with Manual Review and Insights:
- Validate AI-Generated Topics: Regularly review topic clusters and refine them as needed.
- Use Downloadable Reports for Deeper Analysis: Export CSV files of contact reasons and excerpts to further analyze training data.
- Cross-Reference Transcript Data: Use View Transcript to compare AI findings against actual conversation snippets.
Conclusion:
Training Einstein Conversation Mining properly ensures that your AI delivers accurate, actionable insights that improve customer service, sales strategies, and overall business intelligence. By following best practices like defining objectives, refining data quality, leveraging human oversight, and continuously fine-tuning AI models, organizations can maximize the potential of Einstein AI.
Take Your AI Training to the Next Level!
Subscribe to Newsletter
Get our latest blogs directly to your inbox.